VCSEL used in novel photonic neural network
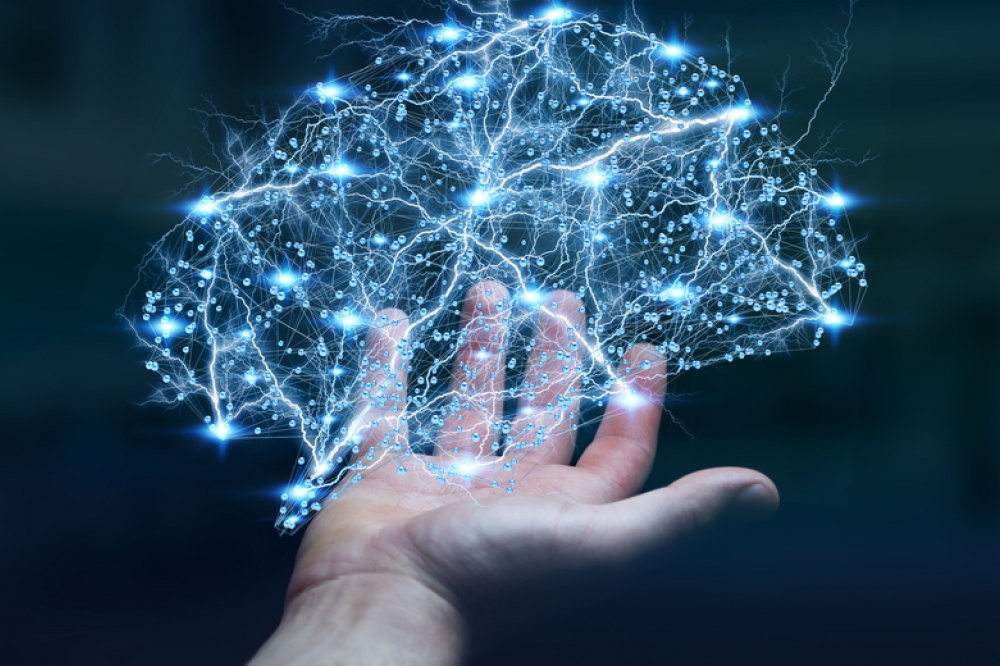
Strathclyde University team achieves high-accuracy classification using a VCSEL and new training method
Photonic neural network systems, which are fast and energy efficient, are helpful for dealing with large amounts of data. To advance photonic brain-like computing technologies, a group of researchers at the University of Strathclyde combined a spike-based neural network with a VCSEL that exhibits spiking neuronal behaviours.
Recently, they presented high-performance photonic spiking neural network operation with lower training requirements and introduced a novel training scheme for getting better results. This research was published Aug. 29 in the journal Intelligent Computing.
The spiking neural network proposed by the authors is based on a hardware-friendly photonic system consisting of just one VCSEL. This proposal represents an improvement on an earlier study by the same authors in which they combined reservoir computing, a powerful method for building photonic neural networks and tackling complex tasks, with a neuromorphic spiking neuron built using a laser of the same type.
In the current paper, the authors accomplished a more challenging classification task and applied an alternative training scheme to improve the speed and efficiency of training while reducing training requirements.
The classification task they tackled is a complex, multivariate and nonlinear problem involving 500 features per data point, and is based on the artificial dataset MADELON. To create the spiking neural network, the authors used an experimental setup that combines the nonlinear spiking dynamics of the laser with an architecture inspired by reservoir computing.
In this architecture, the input data is time-multiplexed. Each time slot represents a virtual neuron in the neural network. The input data is injected into and processed by the laser, and the output is interpreted as a binary node output, either spiking or non-spiking, depending on whether the input data exceeds a certain threshold.
The authors successfully demonstrated the computational power of the photonic spiking neural network with both a traditional least-squares regression training method and their newly proposed “significance” training method. The latter assigns binary weights to nodes based on their overall usefulness and significance.
They report that both methods achieved excellent classification accuracies of over 94 percent, surpassing the benchmark performance in a fraction of the processing time. The accuracies achieved using the new method were 94.4 percent and 95.7 percent, higher than those achieved by the traditional method.
The authors believe that this study could create new possibilities for photonics-based processing systems that operate entirely on optical hardware, enabling them to tackle highly complex tasks with high accuracy, high speed, and energy-efficient operation.
Reference
'Photonic Spiking Neural Networks with Highly Efficient Training Protocols for Ultrafast Neuromorphic Computing Systems' by Dafydd Owen-Newns et al; Intelligent Computing 29 Aug (2023)