Perovskite sensor mimics human eye
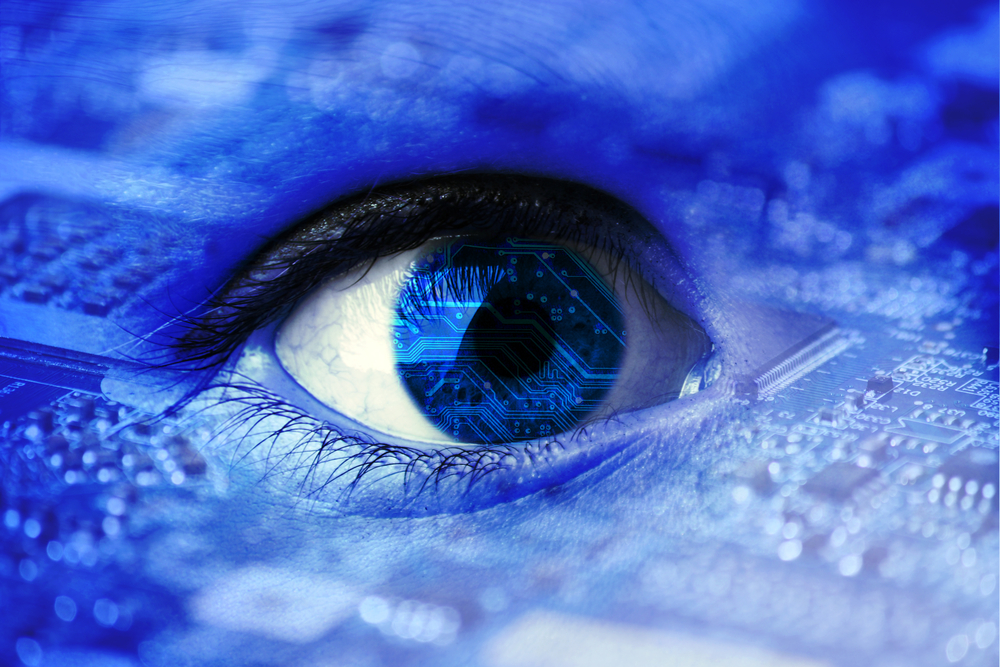
Oregon researchers use ultrathin layers of perovskite which change from strong electrical insulators to strong conductors when placed in light
Researchers at Oregon State University have made a new type of optical sensor built from perovskite. They say the sensor closely mimics the human eye's ability to perceive changes in its visual field. Findings by OSU College of Engineering researcher John Labram and graduate student Cinthya Trujillo Herrera were published in Applied Physics Letters.
Previous attempts to build a human-eye type of device, called a retinomorphic sensor, have relied on software or complex hardware, said Labram, assistant professor of electrical engineering and computer science. But the new sensor's operation is part of its fundamental design, using ultrathin layers of perovskite semiconductors that change from strong electrical insulators to strong conductors when placed in light.
"You can think of it as a single pixel doing something that would currently require a microprocessor," said Labram, who is leading the research effort with support from the National Science Foundation.
The new sensor could be used with neuromorphic computers that will power the next generation of artificial intelligence in applications like self-driving cars, robotics and advanced image recognition, Labram said. Unlike traditional computers, which process information sequentially as a series of instructions, neuromorphic computers are designed to emulate the human brain's massively parallel networks.
"People have tried to replicate this in hardware and have been reasonably successful," Labram said. "However, even though the algorithms and architecture designed to process information are becoming more and more like a human brain, the information these systems receive is still decidedly designed for traditional computers."
In other words: To reach its full potential, a computer that "thinks" more like a human brain needs an image sensor that "sees" more like a human eye.
The eye contains around 100 million photoreceptors. However, the optic nerve only has 1 million connections to the brain. This means that a significant amount of preprocessing and dynamic compression must take place in the retina before the image can be transmitted.
Our vision is particularly well adapted to detect moving objects and is comparatively "less interested" in static images, Labram said. Thus, our optical circuitry gives priority to signals from photoreceptors detecting a change in light intensity - you can demonstrate this yourself by staring at a fixed point until objects in your peripheral vision start to disappear, a phenomenon known as the Troxler effect.
Conventional sensing technologies used in digital cameras and smartphones are better suited to sequential processing, Labram said. Images are scanned across a two-dimensional array of sensors, pixel by pixel, at a set frequency. Each sensor generates a signal with an amplitude that varies directly with the intensity of the light it receives, meaning a static image will result in a more or less constant output voltage from the sensor.
By contrast, the retinomorphic sensor stays relatively quiet under static conditions. It registers a short, sharp signal when it senses a change in illumination, then quickly reverts to its baseline state. This behavior is owed to the unique photoelectric properties of a class of semiconductors known as perovskites, which have shown great promise as next-generation, low-cost solar cell materials.
In Labram's retinomorphic sensor, the perovskite is applied in ultrathin layers, just a few hundred nanometers thick, and functions essentially as a capacitor that varies its capacitance under illumination.
"The way we test it is, basically, we leave it in the dark for a second, then we turn the lights on and just leave them on," he said. "As soon as the light goes on, you get this big voltage spike, then the voltage quickly decays, even though the intensity of the light is constant. And that's what we want."
Although Labram's lab currently can test only one sensor at a time, his team measured a number of devices and developed a numerical model to replicate their behaviour, arriving at what Labram deems "a good match" between theory and experiment.
This enabled the team to simulate an array of retinomorphic sensors to predict how a retinomorphic video camera would respond to input stimulus.
"We can convert video to a set of light intensities and then put that into our simulation," Labram said. "Regions where a higher-voltage output is predicted from the sensor light up, while the lower-voltage regions remain dark. If the camera is relatively static, you can clearly see all the things that are moving respond strongly. This stays reasonably true to the paradigm of optical sensing in mammals."
A simulation using footage of a baseball practice demonstrates the expected results: Players in the infield show up as clearly visible, bright moving objects. Relatively static objects -- the baseball diamond, the bleachers, even the outfielders -- fade into darkness.
An even more striking simulation shows a bird flying into view, then all but disappearing as it stops at an invisible bird feeder. The bird reappears as it takes off. The feeder, set swaying, becomes visible only as it starts to move.
"The good thing is that, with this simulation, we can input any video into one of these arrays and process that information in essentially the same way the human eye would," Labram said. "For example, you can imagine these sensors being used by a robot tracking the motion of objects. Anything static in its field of view would not elicit a response, however a moving object would be registering a high voltage. This would tell the robot immediately where the object was, without any complex image processing."
'A perovskite retinomorphic sensor' by Cinthya Trujillo Herrera and John G. Labram; Appl. Phys. Lett. 117, 233501 (2020)