US team develops AlScN-based AI computing architecture
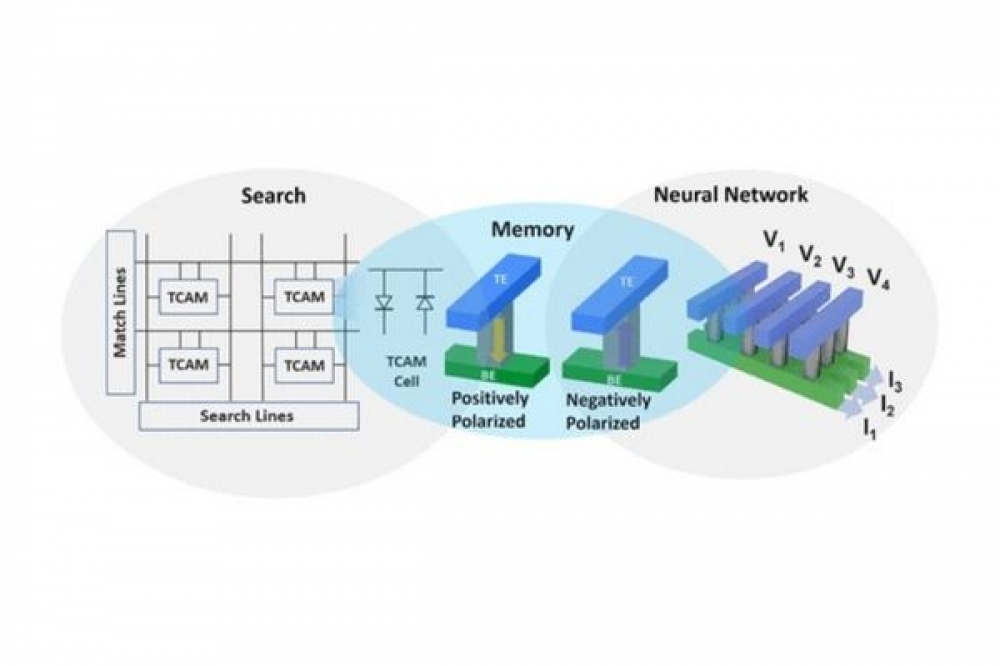
Ferro-diode-based compute-in-memory architecture allows processing and storage to occur in the same place
A team of researchers from the University of Pennsylvania’s School of Engineering and Applied Science, with scientists from Sandia National Laboratories and Brookhaven National Laboratory, has introduced a computing architecture based on ferro-diodes made of AlScN.
Said to be ideal for AI, the AlScN-based compute-in-memory (CIM) architecture allows processing and storage to occur in the same place, eliminating transfer time as well as minimising energy consumption. The work was published Nano Letters.
AlScN has the advantage that it can be deposited at temperatures low enough to be compatible with silicon foundries. “Most ferroelectric materials require much higher temperatures. AlScN’s special properties mean our demonstrated memory devices can go on top of the silicon layer in a vertical hetero-integrated stack," said researcher Troy Olson.
In 2021, the team established the viability of the AlScN as a compute-in-memory powerhouse. Its capacity for miniaturisation, low cost, resource efficiency, ease of manufacture and commercial feasibility demonstrated serious strides in the eyes of research and industry.
In the most recent study , the team observed that their CIM ferrodiode may be able to perform up to 100 times faster than a conventional computing architecture.
Other research in the field has successfully used compute-in-memory architectures to improve performance for AI applications. However, these solutions have been limited, unable to overcome the conflicting trade-off between performance and flexibility. Computing architecture using memristor crossbar arrays, a design that mimics the structure of the human brain to support high-level performance in neural network operations, has also demonstrated admirable speeds.
Yet neural network operations, which use layers of algorithms to interpret data and recognize patterns, are only one of several key categories of data tasks necessary for functional AI. The design is not adaptable enough to offer adequate performance on any other AI data operations.
The Penn team says their ferrodiode design offers groundbreaking flexibility that other compute-in-memory architectures do not. It achieves superior accuracy, performing equally well in not one but three essential data operations that form the foundation of effective AI applications. It supports on-chip storage, or the capacity to hold the enormous amounts of data required for deep learning, parallel search, a function that allows for accurate data filtering and analysis, and matrix multiplication acceleration, the core process of neural network computing.
“It is important to realize that all of the AI computing that is currently done is software-enabled on a silicon hardware architecture designed decades ago,” says Deep Jariwala, who co-led the project. “This is why artificial intelligence as a field has been dominated by computer and software engineers. Fundamentally redesigning hardware for AI is going to be the next big game changer in semiconductors and microelectronics. The direction we are going in now is that of hardware and software co-design.”
'Reconfigurable Compute-In-Memory on Field-Programmable Ferroelectric Diodes' by Xiwen Liu et al; Nano Letters 2022 22 (18)