A new way to find better semiconductors?
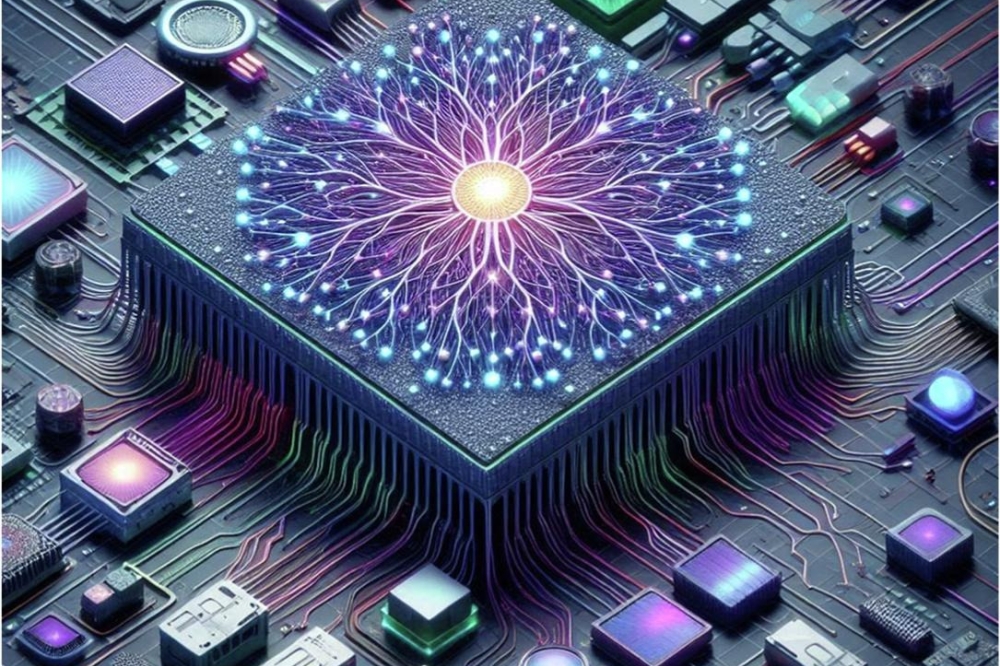
Researchers at Kyoto University have developed a machine learning model integrated with neural networks to predict the band gap of potential semiconductors.
The conventional method of calculating a band gap is expensive and not accurate enough at room temperature, since it is based on a material's properties at absolute zero. "Our model enables prediction based solely on the composition of a compound," says corresponding author Katsuaki Tanabe.
The research team used data from almost 2,000 semiconductor materials tested on six different neural networks. They found that the incorporation of conditional generative adversarial networks, or CGAN, and message passing neural networks, or MPNN, contributed significantly to an improvement in forecast accuracy.
They published their results in the paper 'Neural network ensembles for band gap prediction ' in Computational Materials Science.
The resulting model has achieved the highest prediction accuracy among existing models that have been developed for the same purpose, according to the researchers.
"The computational load of the ensemble learning model is light and can be performed within a few hours on a typical laptop PC," continues Tanabe. "And we can confidently say that this method enables fast and highly accurate forecasting."
Nevertheless, the higher the accuracy of machine learning models, the murkier their internal mechanisms become. Although they are powerful for ad hoc calculations and predictions, they are not versatile or scalable, so more work is required.
"We are also developing other ways of interpreting the correlation between the properties of various materials and band gaps," adds Tanabe, who believes that ensemble models using neural networks are promising for developing a new generation of semiconductors.
Reference
T. Masuda and K. Tanabe. Computational Materials Science, Volume 246 (2025)
Secure Your Hydrogen Supply
A study supply of high-purity hydrogen is critical to semiconductor fabrication. Supply chain interruptions are challenging manufacturers, leading to production slowdowns and stoppages. On-site hydrogen generation offers a scalable alternative for new and existing fabs, freeing the operator from dependence on delivered gas.Plant managers understand the critical role that hydrogen plays in semiconductor fabrication. That important job includes crystal growth, carrier gas, wafer annealing, and in the emerging Extreme UV Lithography (EUV) that will enable new generations of devices. As the vast need for semiconductors grows across all sectors of world economies, so does the need for high-purity hydrogen.
Take control with Nel on-site hydrogen generation.
Read more